Introduction
Currently, fewer than one in twenty prospective loan applicants who start the application process on Acme Co's website (not their real name) get a loan. Following up with every applicant is expensive and unnecessary. My goal was to predict which applicants are good candidates so Acme Co can follow up with the most likely applicants.
The Data
I worked with the data of approximately 19,000 candidates. Instead predicting loans, I predicted who would lock a rate. Locking in a rate reliably leads to a successful loan, and is an earlier stage in the process.
Candidates can apply for loans to both purchase and refinance a home. "Purchase" is a newer category; there are fewer applicants and the lock rate is down about 1%. "Refinance" has a longer history and a higher lock rate.
A Baseline Model
When a candidate applies online, they go through a multi-step process. Most candidates don't make it to the end, but if they do, they are significantly (and unsurprisingly) more likely to lock. As a very simple baseline I predicted that any applicant who reached the fifth stage would lock.
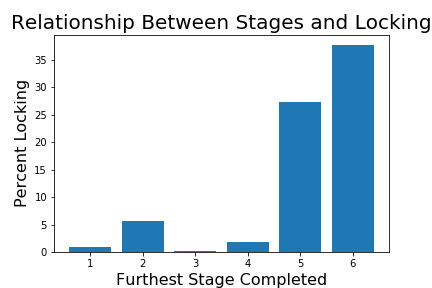
This model does very well, with 92.6% recall and 21.2% precision. Very roughly speaking, this means that using this model, Acme Loans can expect that about a fifth of the people they call will lock, and that they will only miss out on 7.5% of potential lockers.
Can I do better?
My Model
Yes and no.
My best model had a nearly the same scores as the baseline model, so you may be tempted to conclue that they are the same. But, my model allows for more nuance than the baseline model. Recal that the baseline model simply predicted "yes" whenever a user reached at least stage 5. Following the baseline model, Acme Co would never bother calling anyone from the first four stages. This ignores that fact that 20% of all successful applicants stopped before stage 5, and of users who reached stage 5, less than half lock.
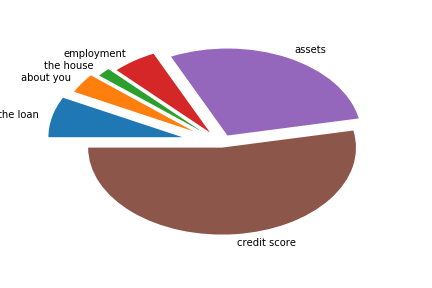
My model allows Acme Co to be more discerning in who they call. It can help identify users who stop early but none the less could be good candidates. And it helps eliminate candidates who aren't likely to lock despite reaching the last stages. It also helps compare two users and identify which of the two is more likely to lock.